Ankylosing spondylitis (AS) is the second most common type of inflammatory arthritis, often affecting teenagers and young adults. Symptoms of AS can include back pain, stiffness, joint inflammation (arthritis), inflammation where tendons attach to bones (enthesitis), and fatigue. Over time, these symptoms can lead to spinal fusion, which significantly affects quality of life, particularly in young people.
Unfortunately, diagnosing AS can be a lengthy process, taking up to ten years from the onset of symptoms and usually requiring X-rays. The slow progression of the condition, coupled with the lack of a definitive test, contributes to these delays.
However, early detection of the condition can make a tremendous difference, halting the degenerative process and preserving a good quality of life for those affected.
Read more:
Unexplained lower back pain? It could be ankylosing spondylitis
Our study explored the potential of using routinely collected healthcare data from GPs and hospitals, combined with advanced machine learning techniques, to identify AS at an earlier stage. Machine learning involves using algorithms to analyse sample data, enabling predictions and decisions without explicit programming.
We analysed data separately for men and women, and our findings could transform the way in which GPs detect and diagnose AS.
A valuable tool
To conduct our study, we used anonymous data from a national data repository at Swansea University Medical School. Patients with AS were identified and matched with people with no record of a diagnosis.
Our analysis of this data found that factors such as lower back pain, uveitis (inflammation of the middle layer of the eye), and use of non-steroidal anti-inflammatory drugs before the age of 20 were factors associated with an increased risk of developing AS in men.
In contrast, our model revealed that women tend to experience AS symptoms at a later age, and often rely on multiple pain relief medications compared with men. This possibly indicates a higher likelihood of misdiagnosis of the condition in women.
Machine learning is a valuable tool for profiling and understanding the characteristics of people who are likely to develop AS. It performs well in test data sets with artificially high prevalence rates.
However, when applied to the general population in GPs and hospitals, where AS is rare, even the best model can only achieve a low positive predictive value of 1.4%. (That’s the probability that following a positive test result, the individual will truly have AS.)
So, using multiple models over time may be necessary to narrow down the population and improve this predictive value, which would result in a faster AS diagnosis.
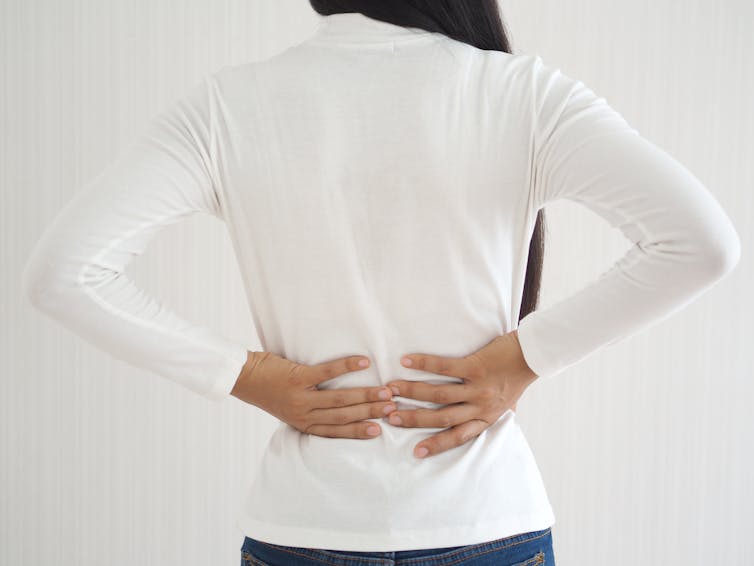
jaojormami/Shutterstock
Acknowledge the challenges too
Machine learning techniques have tremendous potential to improve patient care. But it is also crucial to acknowledge the challenges associated with using these techniques effectively.
These models depend on high-quality data that is diverse and comprehensive to produce reliable, accurate results. But healthcare data can be limited due to privacy concerns, data sensitivity and lack of standardisation. These limitations may therefore compromise the accuracy and reliability of the models.
It’s important to acknowledge that machine learning in relation to this topic is still in its infancy. To develop this further, we will need to gather more detailed data to improve prediction rates and clinical usefulness.
Read more:
From a ‘deranged’ provocateur to IBM’s failed AI superproject: the controversial story of how data has transformed healthcare
But our study demonstrates the enormous potential that machine learning has to help identify people with AS and better understand their diagnostic journeys through the health system.
We know that the early detection and diagnosis of AS is crucial to secure the best outcomes for patients. We believe machine learning could help with this. It could also empower GPs, helping them to detect and refer patients more effectively and efficiently.