[ad_1]
Working pipeline of building up an AI-based detector
To apply the AI-based detection method to the automatic identification of leukocytes, the working pipeline of constructing a deep learning-based detector consists of 4 stages: (1) data preprocessing; (2) model training; (3) inference; and (4) evaluation.
Data preprocessing
The proposed leukocyte dataset is preprocessed in two different data formats using the dataset conversion toolbox developed by the authors. The processed dataset includes ground truth labels and split subsets in VOC and COCO format. The dataset in two formats allows us to be easily input into and trained with popular machine learning methods. The dataset also provides two sizes for all leukocyte images: (1) original size of 3264 × 3264, and (2) reduced size of 600 × 600 (mini size). The data in the former larger size can be used for error analysis, visual inspection or confirmation, and even further investigation. The data in the latter size can be used for model training, which helps to save a lot of CPU overhead time.
Deep learning models
The deep learning model35,36, i.e., convolutional neural networks, is a kind of statistical machine learning models that use convolutional operations to process input data and predict targets in a feedforward fashion. It is proven that deep learning models are capable in fitting information between different data domains. In the leukocyte detectors, we can use deep learning models to fit the medical images onto the leukocyte types and locations in the images.
A typical deep learning-based detector generally consists of two main parts: a feature extraction backbone and a regression head. The feature extraction backbones compute deep features from the image by feedforwarding the information flow through multiple convolutional layers. These deep features are vectors in the same length. The subsequent regression heads project the deep features from previous stage onto the detection output, i.e., confidence values, locations, and types. A key design requirement for the backbone networks is the isometric mapping. Namely, the backbone networks should be able to extract deep features that maintain similar distances in feature space with certain distance metric as the visual distances by human perception. The R-CNN based models37,38,39,40 share similar backbones, while FSAF41 and FCOS42 used Retinanet as their backbone. The regression heads for different models generally have diverse structures or complex designs, but the aim of design is the same: to better use deep features and learn the distributions of the bounding boxes and the types of target objects.
By the different structures of regression heads (with or without region proposal components), the model can be further classified into one-stage detectors41,42 or two-stage detectors37,38,39,40. In general, the one-stage detectors are running faster than the two-stage detectors, while two-stage detectors, which are more complex in general, generate more accurate predictions.
Model training
Using the training set, we build a deep learning-based detector with leukocyte recognition capability. Training samples with ground truth labels are iteratively input into the training algorithm in mini-batches. The models output the predicted results, compared with the ground truth labels. The training process can be stopped when the training loss curve becomes smooth, and the loss value no longer decreases in training. Finally, the internal parameters of the trained models are fixed and can be used in detecting leukocytes in new image samples from clinical practice. We set the training hyper-parameters the same to make the training process consistent for the six models.
Inference
In the inference stage, the detectors judge new image samples they have never seen before. New image samples collected from the clinical practice are rescaled to the input size for the trained detectors. The inference output from the leukocyte detectors includes three data items, the predicted types of leukocytes, the confidence values, and the locations of the leukocytes in the image. In our proposed method, the ensemble scheme is based on the trained detectors, optimizes the results from the results of other detectors, then outputs the final results.
Evaluation
In the evaluation stage, the inferred results are evaluated with multiple metrics, and the model’s performance is analyzed with different criteria. Evaluating metrics include mAP and mAR under the different values of IoU. Besides, we record executive performance, i.e., the model’s size and the inference speed in frame per second (FPS), which helps evaluate whether the detectors are suitable or feasible to put into practice. As for accuracy, we emphasize the mAP because it is a popular and proven performance indicator in object detection. To further analyze the classification capability, we measure the average precision for each type of leukocyte.
Ensemble scheme for deep learning-based models
In machine learning, an ensemble model is a voting scheme that combines the predictions from multiple other models. The ensemble model’s work is similar to the collective judgment by a medical expert panel in diagnosing a complicated case. The advantage of an ensemble model is that the final results are more stable for complex samples and potentially more accurate in quantitative evaluation. On the other hand, however, it may cost more computational time in inference. In this work, we integrate the ensemble scheme into the inference stage and evaluate its results. Ensemble linearly combines the bounding boxes of leukocytes with the corresponding confidences as the weights43. Given a list of \(N\) overlapping predicted bounding boxes \(\left\{ \overset\lower0.5em\hbox$\smash\scriptscriptstyle\rightharpoonup$ L ^i = \left[ x_1^i ,\text~y_1^i ,x_2^i ,y_2^i \right] \right\}\) for leukocytes and the corresponding confidence values \(\C^i\\) from \(T\) models. \(T\) is the number of models which make most predictions on the leukocyte to be the same type. The number \(T\) is less than or equal to \(N\), because of possible false negative detection; and greater than \(N/2\) because these might be some false positive detection. The averaged bounding box \(\overset\lower0.5em\hbox$\smash\scriptscriptstyle\rightharpoonup$ L ^\prime = \left[ x_1^\prime ,\text~y_1^\prime ,x_2^\prime ,y_2^\prime \right]\) and the updated confidence values \(C^\mathrm^\prime\) are given as:
$$\left[ x^\prime_1 ,y^\prime_1 ,x^\prime_2 ,y^\prime_2 \right] = \frac{{\sum\nolimits_1^N \left( C^i \cdot \left[ x_1^i ,y_1^i ,x_2^i ,y_2^i \right] \right) }}\sum\nolimits_1^N C^i ,$$
and
$$C^\prime = \frac{\sum\nolimits_1^N C^i }T.$$
The former equation computes the averaged location by considering the confidences of predictions from different models. A model needs to be more confident about its prediction so that its vote is more potent in influencing the final result. The latter equation suggests that the updated confidence values are averaged over the number of models rather than the number of predictions. In this way, if some models deem that the area has no leukocyte, like abstention, the updated confidence values will be lowered.
A simple illustrative example is shown in Fig. 1. Suppose we have trained six models and five out of six output the 5 detections as D1, D2, D3, D4, and D5 in the left image in Fig. 1. Each Di contains a bounding box location \(\overset\lower0.5em\hbox$\smash\scriptscriptstyle\rightharpoonup$ L ^i\), a confidence value \(C^i\), and a type label Pi. In the ensemble process, if the number of overlapping detections for a possible object is less \(N/2\), these detections will be discarded in the first place. Thus, in this example, D1 is ruled out, leaving D1, D2, D3, and D4 for further processing. Three (D1, D2, D3) out of the four detections predict leukocyte as neutrophil granulocyte, and then the ensemble scheme judges the type of ensemble prediction E as the same as that of the majority. The ensemble scheme subsequently calculates the confidence value and the bounding by computing the corresponding mean values.
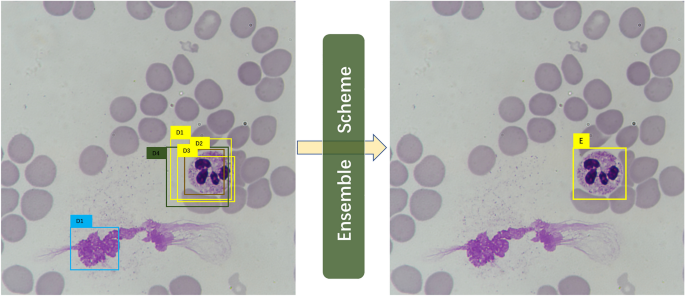
An illustrative example of the ensemble scheme. The raw detections are given in the left image, and the right image is the result after ensemble.
Implementation details
The experiments are implemented on a regular workstation computer with Intel Core i5-8600 CPU, 16 GB RAM, and an Nvidia TITAN Xp Graphics Card with 12 GB graphic memory. The software environments are based on Ubuntu 18.04 OS, and training is carried out on PyTorch 1.7.0 (https://pytorch.org/) and mmdetection 2.6.0 codebases (https://github.com/open-mmlab/mmdetection). The training epoch is set to 16, which is high enough for training convergence for the detectors. Setting this relatively excessive number of epochs is to ensure the models can approach the optimal state and avoid underfitting. The recorded training time for the models is around two hours.
The models can be efficiently trained in such a short time because of the use of pre-trained backbone networks and fine-tuning techniques. The fine-tuning technique allows the model to shift its intelligence from recognizing generic objects to detecting leukocytes. The pre-trained weights are trained parameters from a deep learning-based model (Resnet-5044) in classifying objects in the ImageNet dataset or detecting objects in the MS-COCO dataset. To fine-tune a model, we freeze the parameters in the low-level filters, which compute basic image texture features. On the other, high-level parameters, which are, for a reason, the structural information, are gradually updated by the backpropagation approach. This way, it adjusts the high-level parameters in pre-trained weights to our leukocyte detection task.
When training detectors, we used stochastic gradient descent (SGD) as the optimizer for the model parameter update. The key hyper-parameters, i.e., the learning rate, momentum, and weight decay coefficient of SGD, are set to 0.01, 0.9, and 0.0001, respectively. Other detailed configurations for the detector architecture are defaults except for these settings.
Data augmentation technique is employed in model training. Data augmentation is an online process that dynamically generates variants of training samples before feeding them into the model, following the sampling of the mini-batch of training data from the training set. Supplementary Table 1 shows a transformation list of data augmentation used in our implementation. The transforms are composed of an occurrence probability for each transform.
Ethical approval
All blood smears involved in this study are historical samples. Since only blood smears from patients are photographed, the approval of the institutional review board is not required.
[ad_2]
Source link